Away Team Droid Tech: Robotic Planning Under Uncertainty in Spatiotemporal Environments in Expeditionary Science
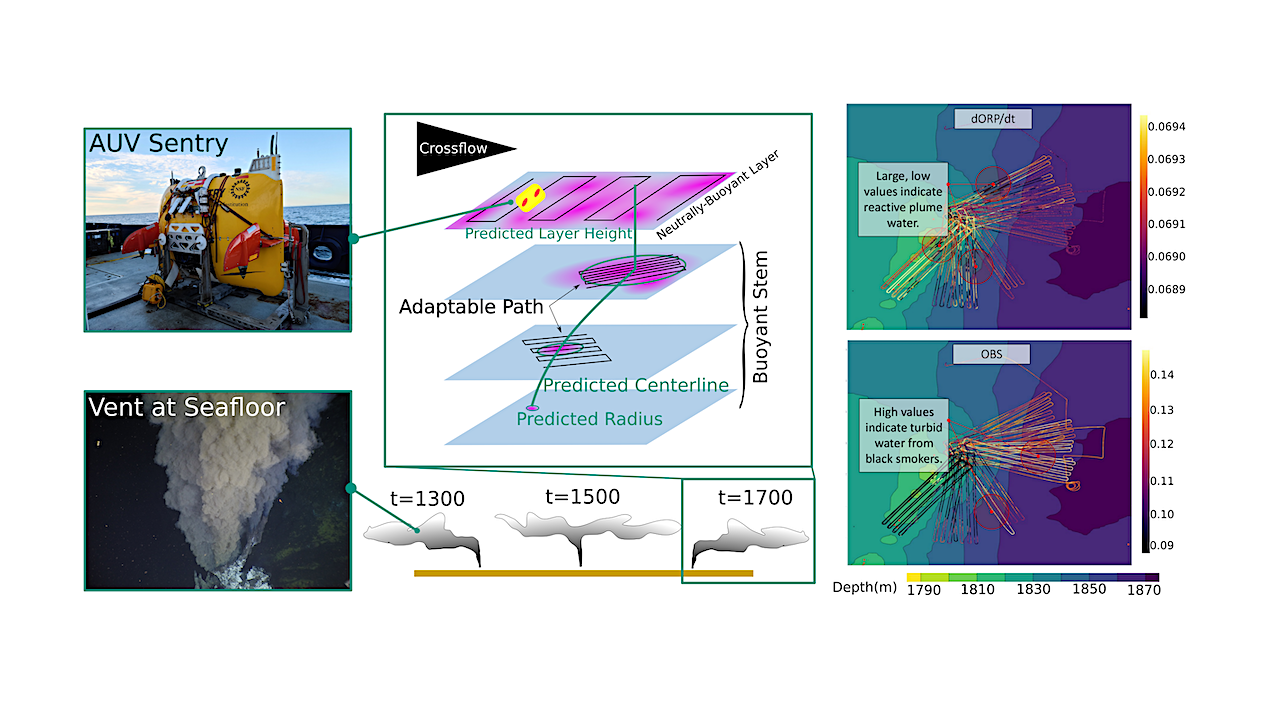
In the expeditionary sciences, spatiotemporally varying environments — hydrothermal plumes, algal blooms, lava flows, or animal migrations — are ubiquitous. Mobile robots are uniquely well-suited to study these dynamic, mesoscale natural environments.
We formalize expeditionary science as a sequential decision-making problem, modeled using the language of partially-observable Markov decision processes (POMDPs). Solving the expeditionary science POMDP under real-world constraints requires efficient probabilistic modeling and decision-making in problems with complex dynamics and observational models.
Previous work in informative path planning, adaptive sampling, and experimental design have shown compelling results, largely in static environments, using data-driven models and information-based rewards.
However, these methodologies do not trivially extend to expeditionary science in spatiotemporal environments: they generally do not make use of scientific knowledge such as equations of state dynamics, they focus on information gathering as opposed to scientific task execution, and they make use of decision-making approaches that scale poorly to large, continuous problems with long planning horizons and real-time operational constraints.
In this work, we discuss these and other challenges related to probabilistic modeling and decision-making in expeditionary science, and present some of our preliminary work that addresses these gaps. We ground our results in a real expeditionary science deployment of an autonomous underwater vehicle (AUV) in the deep ocean for hydrothermal vent discovery and characterization.
Our concluding thoughts highlight remaining work to be done, and the challenges that merit consideration by the reinforcement learning and decision-making community.
Victoria Preston, Genevieve Flaspohler, Anna P. M. Michel, John W. Fisher III, Nicholas Roy
Comments: 5 pages, 1 figure, as submitted to The Multi-disciplinary Conference on Reinforcement Learning and Decision Making
Subjects: Robotics (cs.RO)
Cite as: arXiv:2206.01364 [cs.RO] (or arXiv:2206.01364v1 [cs.RO] for this version)
https://doi.org/10.48550/arXiv.2206.01364
Focus to learn more
Submission history
From: Victoria Preston
[v1] Fri, 3 Jun 2022 02:04:15 UTC (8,121 KB)
https://arxiv.org/abs/2206.01364
Astrobiology,