Machine Vision and Deep Learning for Classification of Radio SETI Signals
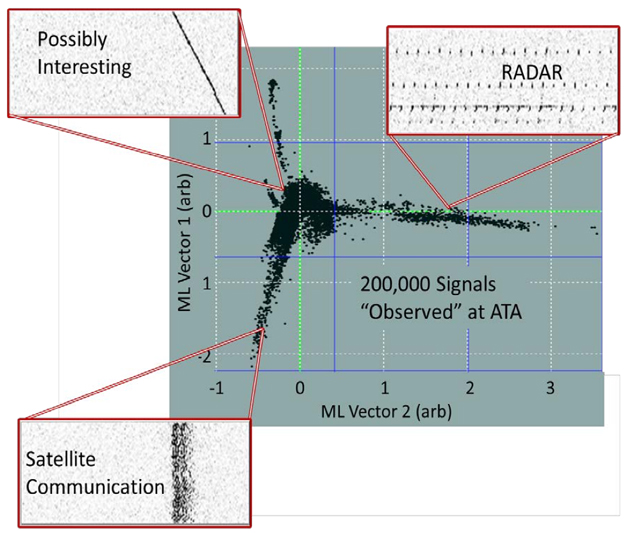
We apply classical machine vision and machine deep learning methods to prototype signal classifiers for the search for extraterrestrial intelligence. Our novel approach uses two-dimensional spectrograms of measured and simulated radio signals bearing the imprint of a technological origin.
The studies are performed using archived narrow-band signal data captured from real-time SETI observations with the Allen Telescope Array and a set of digitally simulated signals designed to mimic real observed signals. By treating the 2D spectrogram as an image, we show that high quality parametric and non-parametric classifiers based on automated visual analysis can achieve high levels of discrimination and accuracy, as well as low false-positive rates. The (real) archived data were subjected to numerous feature-extraction algorithms based on the vertical and horizontal image moments and Huff transforms to simulate feature rotation. The most successful algorithm used a two-step process where the image was first filtered with a rotation, scale and shift-invariant affine transform followed by a simple correlation with a previously defined set of labeled prototype examples.
The real data often contained multiple signals and signal ghosts, so we performed our non-parametric evaluation using a simpler and more controlled dataset produced by simulation of complex-valued voltage data with properties similar to the observed prototypes. The most successful non-parametric classifier employed a wide residual (convolutional) neural network based on pre-existing classifiers in current use for object detection in ordinary photographs. These results are relevant to a wide variety of research domains that already employ spectrogram analysis from time-domain astronomy to observations of earthquakes to animal vocalization analysis.
G. R. Harp, Jon Richards, Seth Shostak Jill C. Tarter, Graham Mackintosh, Jeffrey D. Scargle, Chris Henze, Bron Nelson, G. A. Cox, S. Egly, S. Vinodababu, J. Voien
(Submitted on 6 Feb 2019)
Comments: 31 pages, 7 figures, 4 tables, submitted to Astronomical Journal
Subjects: Instrumentation and Methods for Astrophysics (astro-ph.IM); Earth and Planetary Astrophysics (astro-ph.EP)
Cite as: arXiv:1902.02426 [astro-ph.IM] (or arXiv:1902.02426v1 [astro-ph.IM] for this version)
Submission history
From: Gerald Harp Ph.D.
[v1] Wed, 6 Feb 2019 23:08:22 UTC (1,266 KB)
https://arxiv.org/abs/1902.02426
Astrobiology