Can Close-In Exoplanets Form By Pebble Accretion?
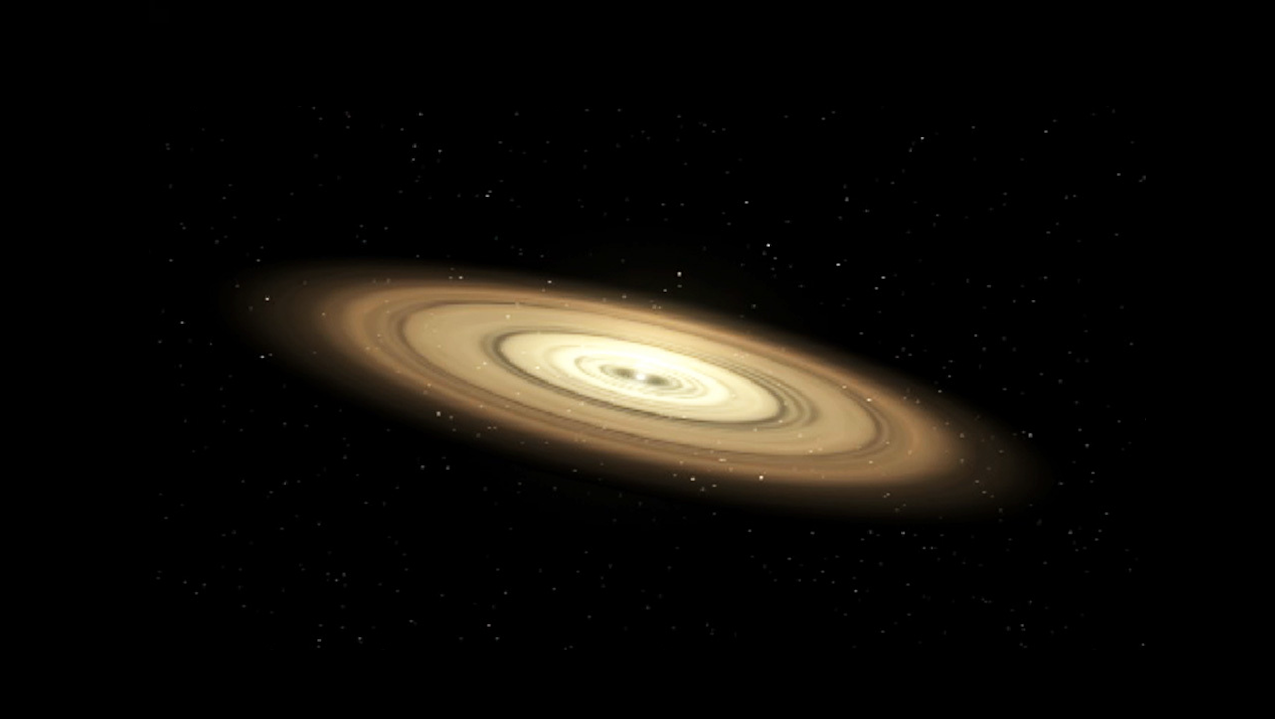
Pebble accretion is the leading theory for the formation of exoplanets more massive than the Earth.
Many parameters influence planet growth in the pebble accretion models. In this paper, we study the influence of pebble fragmentation velocity, turbulence strength, stellar metallicity, stellar mass, and planet location on the growth of planets located within 1 au from their parent stars.
Analysing the close-in planets from NASA’s Exoplanet Archive, we find that the turbulence strength influences planet growth more than the pebble fragmentation velocity does.
Planets orbiting stars with higher metallicity have an overall higher probability of reaching their pebble isolation mass than those orbiting lower metallicity stars, but the impact of metallicity is not as high as that of stellar mass, orbital separation, and most importantly disc turbulence.
Jayashree Narayan, Joanna Drazkowska, Vignesh Vaikundaraman
Comments: 10 pages, 7 figures, Accepted for publication in MNRAS
Subjects: Earth and Planetary Astrophysics (astro-ph.EP)
Cite as: arXiv:2505.00090 [astro-ph.EP] (or arXiv:2505.00090v1 [astro-ph.EP] for this version)
https://doi.org/10.48550/arXiv.2505.00090
Focus to learn more
Submission history
From: Jayashree Narayan
[v1] Wed, 30 Apr 2025 18:01:01 UTC (457 KB)
https://arxiv.org/abs/2505.00090
Astrobiology,