MODEL&CO: Exoplanet Detection In Angular Differential Imaging By Learning Across Multiple Observations
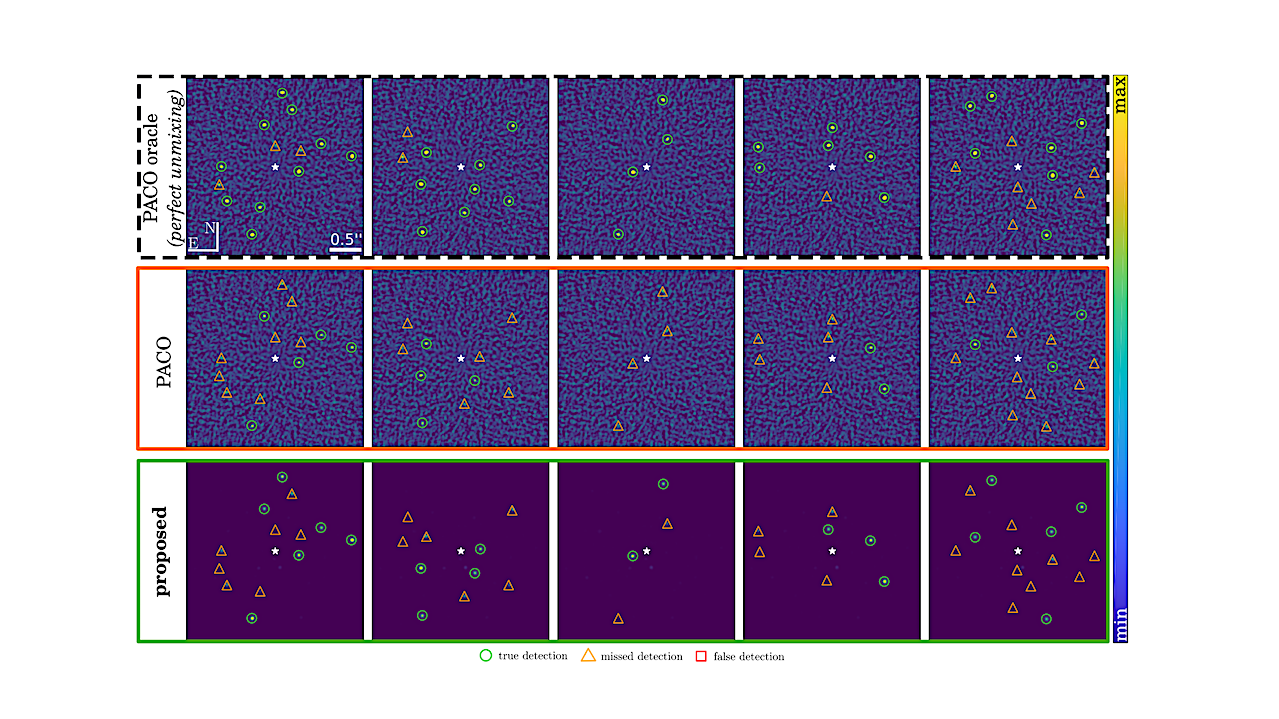
Direct imaging of exoplanets is particularly challenging due to the high contrast between the planet and the star luminosities, and their small angular separation. In addition to tailored instrumental facilities implementing adaptive optics and coronagraphy, post-processing methods combining several images recorded in pupil tracking mode are needed to attenuate the nuisances corrupting the signals of interest.
Most of these post-processing methods build a model of the nuisances from the target observations themselves, resulting in strongly limited detection sensitivity at short angular separations due to the lack of angular diversity. To address this issue, we propose to build the nuisance model from an archive of multiple observations by leveraging supervised deep learning techniques.
The proposed approach casts the detection problem as a reconstruction task and captures the structure of the nuisance from two complementary representations of the data. Unlike methods inspired by reference differential imaging, the proposed model is highly non-linear and does not resort to explicit image-to-image similarity measurements and subtractions. The proposed approach also encompasses statistical modeling of learnable spatial features.
The latter is beneficial to improve both the detection sensitivity and the robustness against heterogeneous data. We apply the proposed algorithm to several datasets from the VLT/SPHERE instrument, and demonstrate a superior precision-recall trade-off compared to the PACO algorithm.
Interestingly, the gain is especially important when the diversity induced by ADI is the most limited, thus supporting the ability of the proposed approach to learn information across multiple observations.
Théo Bodrito, Olivier Flasseur, Julien Mairal, Jean Ponce, Maud Langlois, Anne-Marie Lagrange
Subjects: Instrumentation and Methods for Astrophysics (astro-ph.IM); Earth and Planetary Astrophysics (astro-ph.EP); Computer Vision and Pattern Recognition (cs.CV); Data Analysis, Statistics and Probability (physics.data-an)
Cite as: arXiv:2409.17178 [astro-ph.IM] (or arXiv:2409.17178v1 [astro-ph.IM] for this version)
https://doi.org/10.48550/arXiv.2409.17178
Focus to learn more
Submission history
From: Théo Bodrito
[v1] Mon, 23 Sep 2024 09:22:45 UTC (20,858 KB)
https://arxiv.org/abs/2409.17178
Astrobiology