ExoMiner++ on TESS with Transfer Learning from Kepler: Transit Classification and Vetting Catalog for 2-min Data
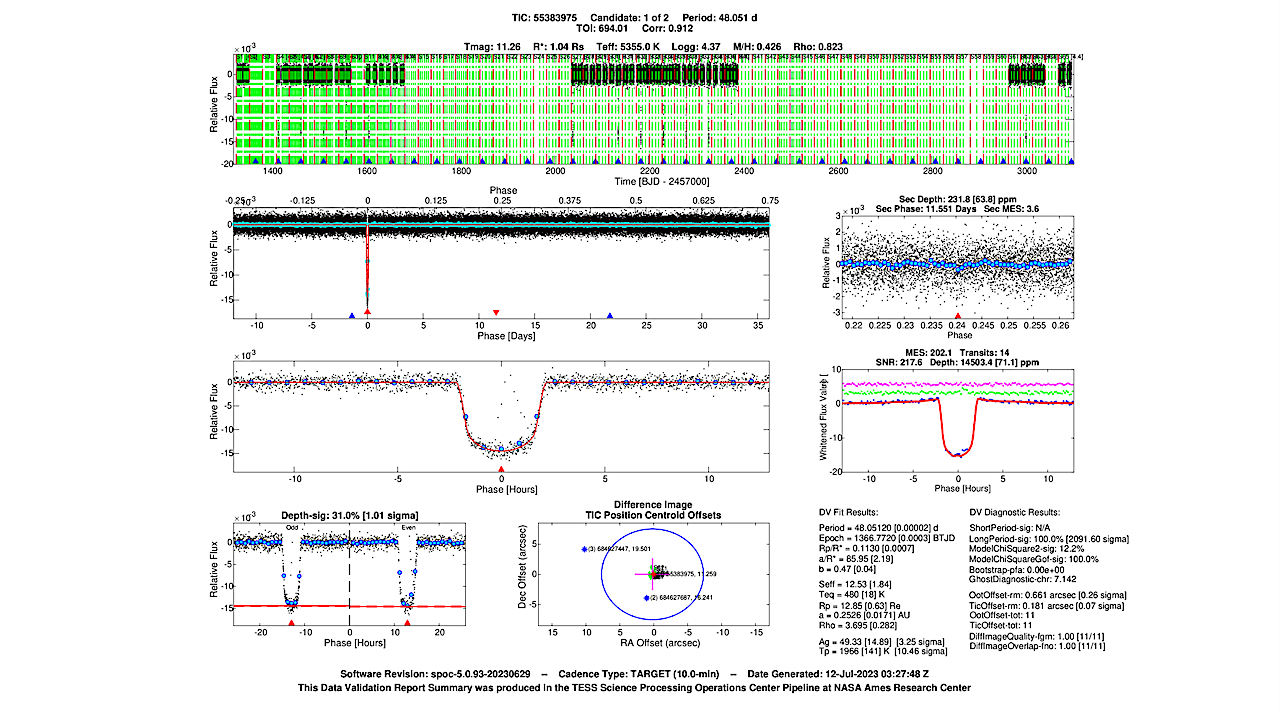
We present ExoMiner++, an enhanced deep learning model that builds on the success of ExoMiner to improve transit signal classification in 2-minute TESS data. ExoMiner++ incorporates additional diagnostic inputs, including periodogram, flux trend, difference image, unfolded flux, and spacecraft attitude control data, all of which are crucial for effectively distinguishing transit signals from more challenging sources of false positives.
To further enhance performance, we leverage transfer learning from high-quality labeled data from the Kepler space telescope, mitigating the impact of TESS’s noisier and more ambiguous labels. ExoMiner++ achieves high accuracy across various classification and ranking metrics, significantly narrowing the search space for follow-up investigations to confirm new planets.
To serve the exoplanet community, we introduce new TESS catalogs containing ExoMiner++ classifications and confidence scores for each transit signal. Among the 147,568 unlabeled TCEs, ExoMiner++ identifies 7,330 as planet candidates, with the remainder classified as false positives.
These 7,330 planet candidates correspond to 1,868 existing TESS Objects of Interest (TOIs), 69 Community TESS Objects of Interest (CTOIs), and 50 newly introduced CTOIs. 1,797 out of the 2,506 TOIs previously labeled as planet candidates in ExoFOP are classified as planet candidates by ExoMiner++.
This reduction in plausible candidates combined with the excellent ranking quality of ExoMiner++ allows the follow-up efforts to be focused on the most likely candidates, increasing the overall planet yield.
Hamed Valizadegan, Miguel J. S. Martinho, Jon M. Jenkins, Joseph D. Twicken, Douglas A. Caldwell, Patrick Maynard, Hongbo Wei, William Zhong, Charles Yates, Sam Donald, Karen A. Collins, David Latham, Khalid Barkaoui, Perry Berlind, Michael L. Calkins, Kylee Carden, Nikita Chazov, Gilbert A. Esquerdo, Tristan Guillot, Vadim Krushinsky, Grzegorz Nowak, Benjamin V. Rackham, Amaury Triaud, Richard P. Schwarz, Denise Stephens, Chris Stockdale, Jiaqi Wang, Cristilyn N. Watkins, Francis P. Wilkin
Subjects: Earth and Planetary Astrophysics (astro-ph.EP); Instrumentation and Methods for Astrophysics (astro-ph.IM); Machine Learning (cs.LG)
Cite as: arXiv:2502.09790 [astro-ph.EP] (or arXiv:2502.09790v1 [astro-ph.EP] for this version)
https://doi.org/10.48550/arXiv.2502.09790
Focus to learn more
Submission history
From: Hamed Valizadegan
[v1] Thu, 13 Feb 2025 21:53:58 UTC (6,648 KB)
https://arxiv.org/abs/2502.09790
Astrobiology,