Constructing Impactful Machine Learning Research For Astronomy: Best Practices For Researchers And Reviewers
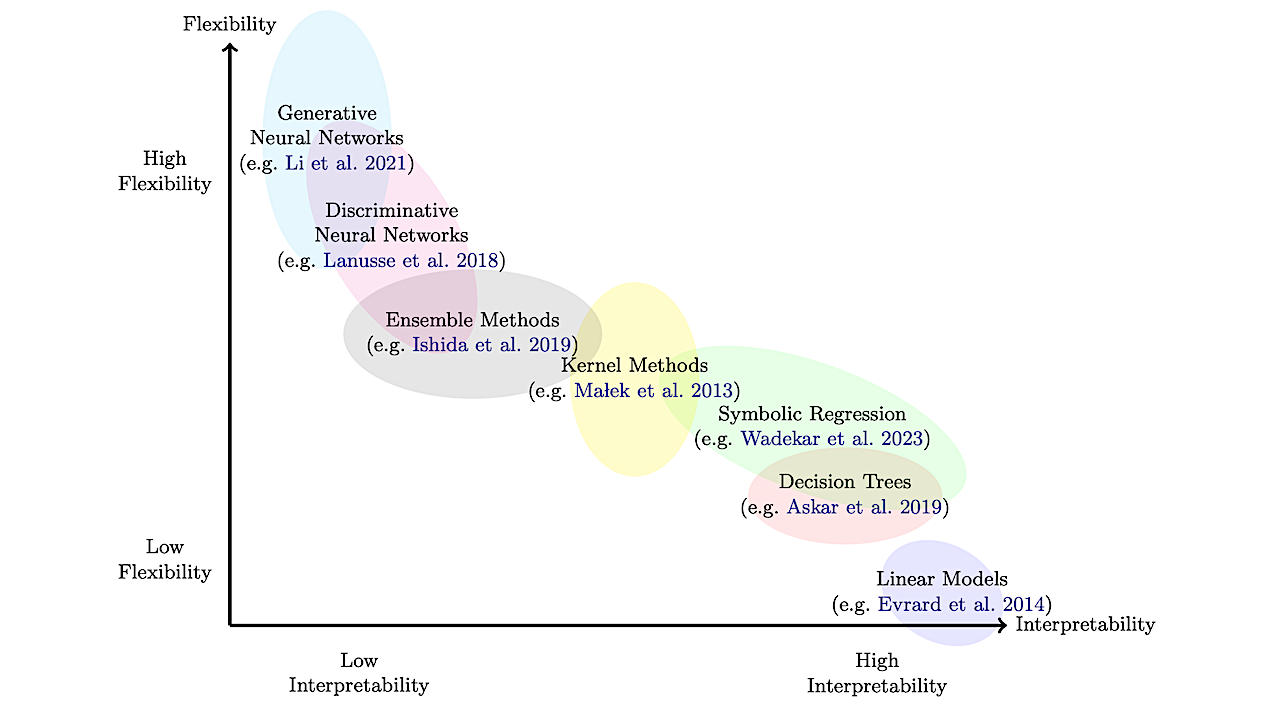
Machine learning has rapidly become a tool of choice for the astronomical community. It is being applied across a wide range of wavelengths and problems, from the classification of transients to neural network emulators of cosmological simulations, and is shifting paradigms about how we generate and report scientific results.
At the same time, this class of method comes with its own set of best practices, challenges, and drawbacks, which, at present, are often reported on incompletely in the astrophysical literature.
With this paper, we aim to provide a primer to the astronomical community, including authors, reviewers, and editors, on how to implement machine learning models and report their results in a way that ensures the accuracy of the results, reproducibility of the findings, and usefulness of the method.
D. Huppenkothen, M. Ntampaka, M. Ho, M. Fouesneau, B. Nord, J. E. G. Peek, M. Walmsley, J. F. Wu, C. Avestruz, T. Buck, M. Brescia, D. P. Finkbeiner, A. D. Goulding, T. Kacprzak, P. Melchior, M. Pasquato, N. Ramachandra, Y.-S. Ting, G. van de Ven, S. Villar, V.A. Villar, E. Zinger
Comments: 14 pages, 3 figures; submitted to the Bulletin of the American Astronomical Society
Subjects: Instrumentation and Methods for Astrophysics (astro-ph.IM); Machine Learning (cs.LG)
Cite as: arXiv:2310.12528 [astro-ph.IM] (or arXiv:2310.12528v1 [astro-ph.IM] for this version)
Submission history
From: Daniela Huppenkothen
[v1] Thu, 19 Oct 2023 07:04:36 UTC (130 KB)
https://arxiv.org/abs/2310.12528
Astrobiology, Astronomy, Astrochemisty,