deep PACO: Combining Statistical Models With Deep Learning For Exoplanet Detection And Characterization In Direct Imaging At High Contrast
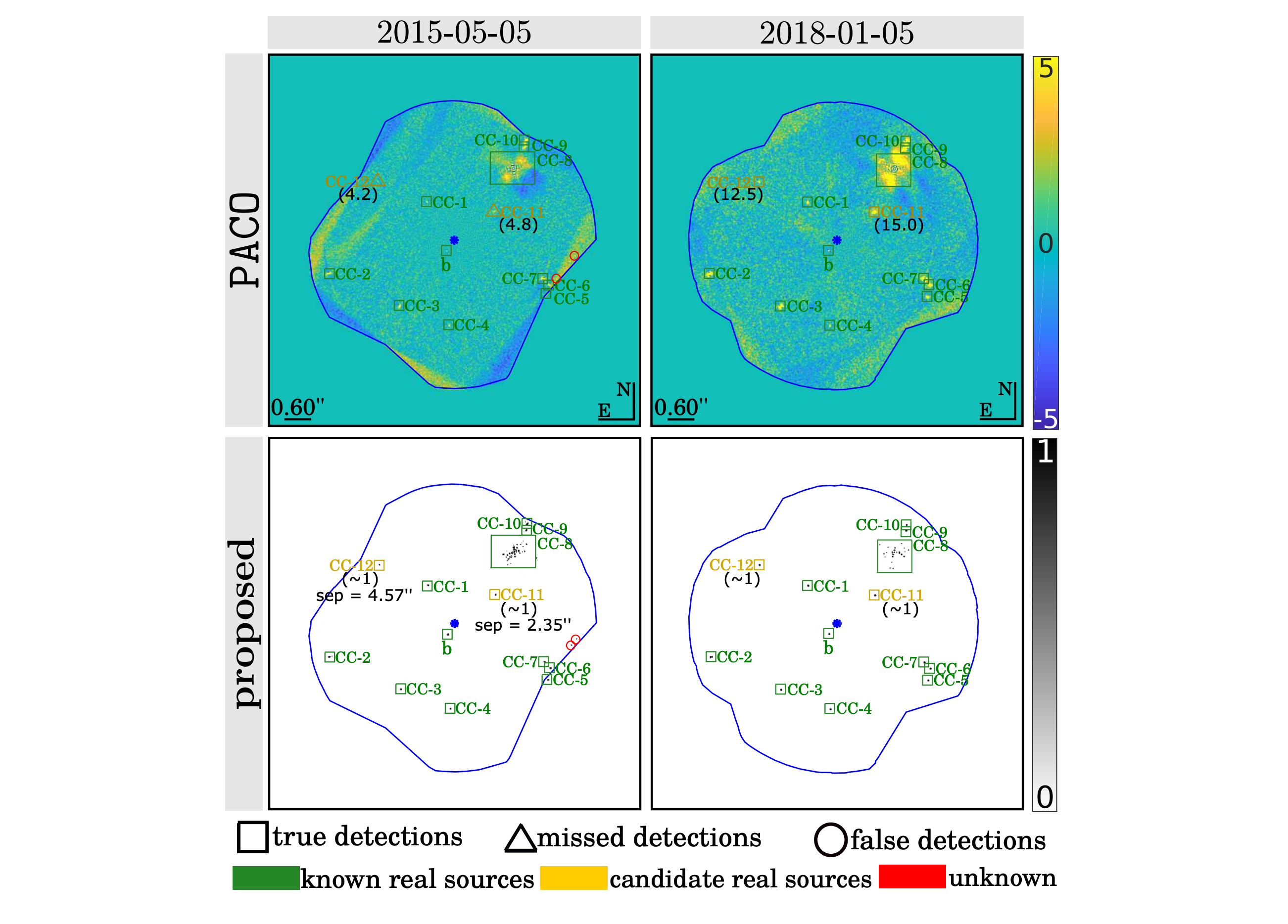
Direct imaging is an active research topic in astronomy for the detection and the characterization of young sub-stellar objects. The very high contrast between the host star and its companions makes the observations particularly challenging.
In this context, post-processing methods combining several images recorded with the pupil tracking mode of telescope are needed. In previous works, we have presented a data-driven algorithm, PACO, capturing locally the spatial correlations of the data with a multi-variate Gaussian model. PACO delivers better detection sensitivity and confidence than the standard post-processing methods of the field.
However, there is room for improvement due to the approximate fidelity of the PACO statistical model to the time evolving observations. In this paper, we propose to combine the statistical model of PACO with supervised deep learning. The data are first pre-processed with the PACO framework to improve the stationarity and the contrast. A convolutional neural network (CNN) is then trained in a supervised fashion to detect the residual signature of synthetic sources. Finally, the trained network delivers a detection map.
The photometry of detected sources is estimated by a second CNN. We apply the proposed approach to several datasets from the VLT/SPHERE instrument. Our results show that its detection stage performs significantly better than baseline methods (cADI, PCA), and leads to a contrast improvement up to half a magnitude compared to PACO. The characterization stage of the proposed method performs on average on par with or better than the comparative algorithms (PCA, PACO) for angular separation above 0.5”.
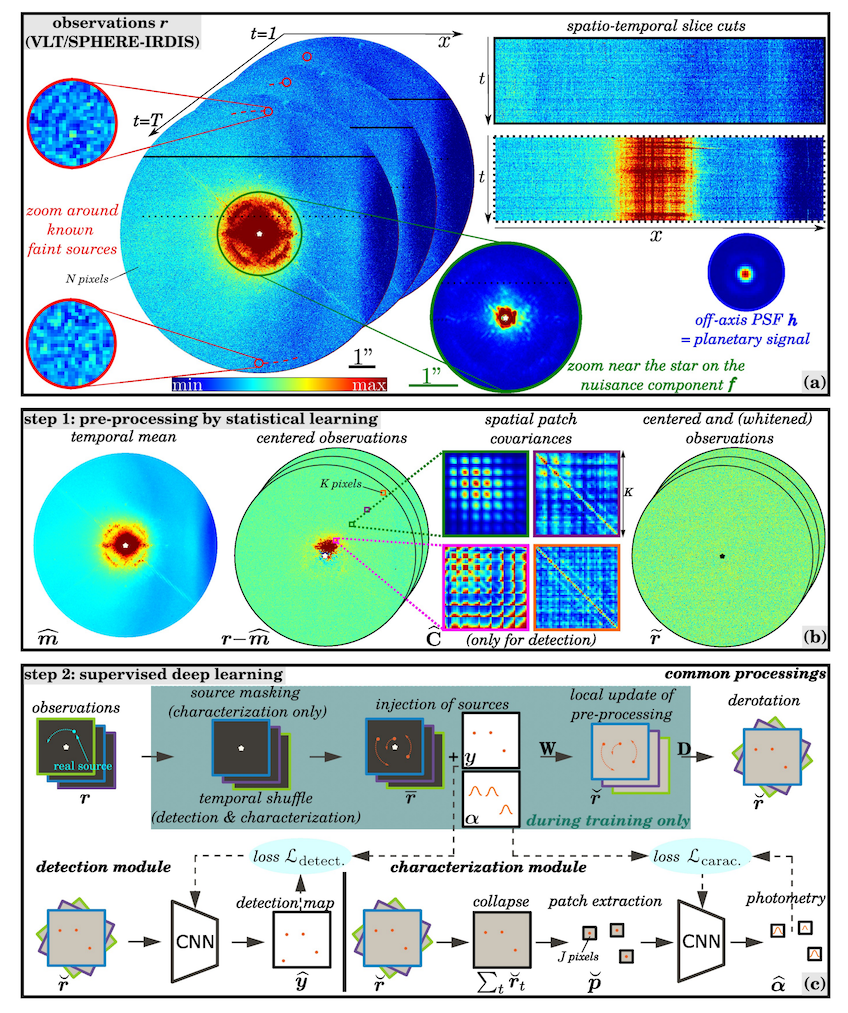
(a) Typical observations đť’“ from the VLT/SPHERE-IRDIS instrument conducted in pupil tracking mode (i.e., with the ADI technique). Zooms around two known background faint sources are displayed in the red circles, a zoom on the nuisance component đť’‡ near the star is highlighted in the green circle, and a view of the sought exoplanetary signal, taking the form of the off-axis PSF đť’‰, is shown in the blue circle. Two spatio-temporal slice cuts along the black solid and dashed lines are shown on the right, as an illustration of the spatial non-stationarity of the correlations of the nuisance. (b) Illustration of the main operations performed during the pre-processing of the proposed algorithm by statistical learning. Examples of estimated spatial covariance matrices are displayed in squares for four regions of interest pickled at about 0.5 (pink), 1.0 (green), 1.5 (purple), and 2.0 (orange) arcsec. (c) Schematic representation of the main operations performed during the detection and characterization steps of the proposed algorithm by supervised deep learning. The first line displays common operations performed for both the detection and the characterization. The left (respectively, the right) part of the second line is for operations applied solely during the detection (respectively, the characterization) step. Dataset: HIP 72192 (2015-05-10), see Sect. 4 for the recording logs. — astro-ph.IM
Olivier Flasseur, Théo Bodrito, Julien Mairal, Jean Ponce, Maud Langlois, Anne-Marie Lagrange
Comments: Submitted to Monthly Notices of the Royal Astronomical Society
Subjects: Instrumentation and Methods for Astrophysics (astro-ph.IM); Data Analysis, Statistics and Probability (physics.data-an)
Cite as: arXiv:2303.02461 [astro-ph.IM] (or arXiv:2303.02461v1 [astro-ph.IM] for this version)
Submission history
From: Olivier Flasseur
[v1] Sat, 4 Mar 2023 17:36:03 UTC (46,189 KB)
https://arxiv.org/abs/2303.02461
Astrobiology