Neural Network Constraints on the Cosmic-Ray Ionization Rate and Other Physical Conditions in NGC 253 with ALCHEMI Measurements of HCN and HNC
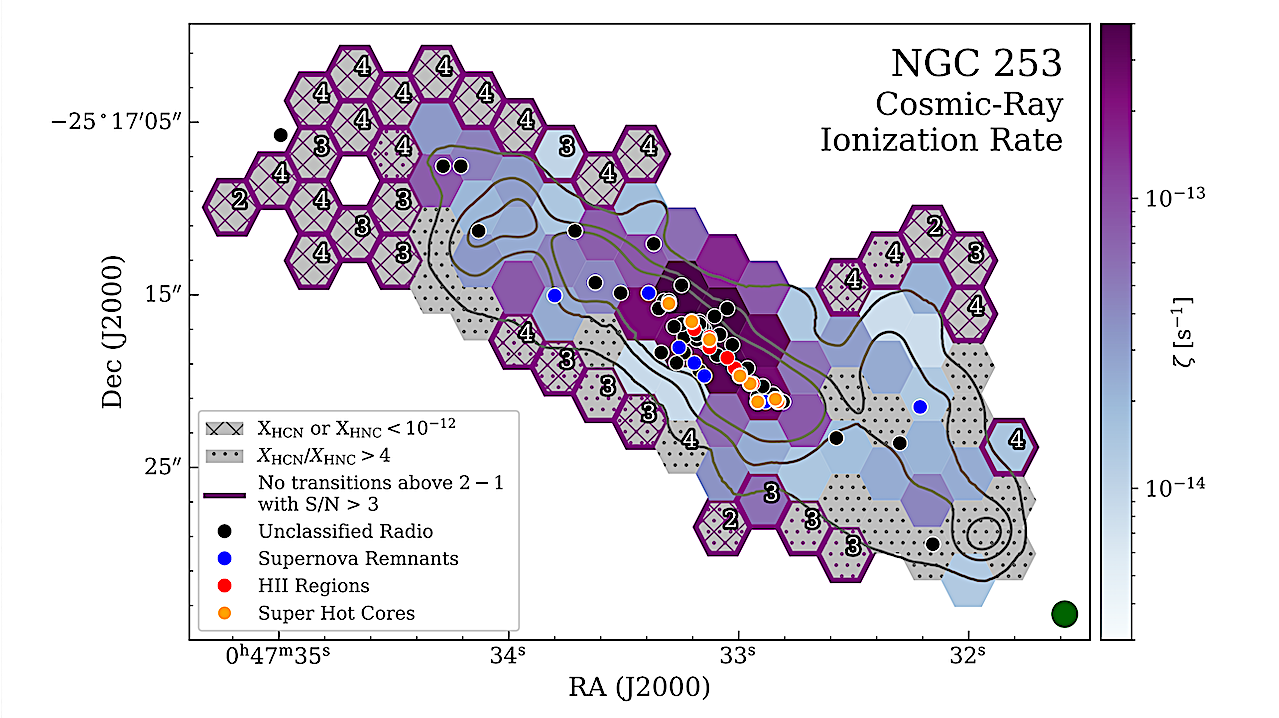
We use a neural network model and ALMA observations of HCN and HNC to constrain the physical conditions, most notably the cosmic-ray ionization rate (CRIR, zeta), in the Central Molecular Zone (CMZ) of the starburst galaxy NGC 253.
Using output from the chemical code UCLCHEM, we train a neural network model to emulate UCLCHEM and derive HCN and HNC molecular abundances from a given set of physical conditions. We combine the neural network with radiative transfer modeling to generate modeled integrated intensities, which we compare to measurements of HCN and HNC from the ALMA Large Program ALCHEMI. Using a Bayesian nested sampling framework, we constrain the CRIR, molecular gas volume and column densities, kinetic temperature, and beam-filling factor across NGC 253’s CMZ.
The neural network model successfully recovers UCLCHEM molecular abundances with about 3 percent error and, when used with our Bayesian inference algorithm, increases the parameter inference speed tenfold. We create images of these physical parameters across NGC 253’s CMZ at 50 pc resolution and find that the CRIR, in addition to the other gas parameters, is spatially variable with zeta a few times 10^{14} s^{-1} at greater than 100 pc from the nucleus, increasing to zeta greater than 10^{-13} s^{-1} at its center.
These inferred CRIRs are consistent within 1 dex with theoretical predictions based on non-thermal emission. Additionally, the high CRIRs estimated in NGC 253’s CMZ can be explained by the large number of cosmic-ray-producing sources as well as a potential suppression of cosmic-ray diffusion near their injection sites.
Erica Behrens, Jeffrey G. Mangum, Serena Viti, Jonathan Holdship, Ko-Yun Huang, Mathilde Bouvier, Joshua Butterworth, Cosima Eibensteiner, Nanase Harada, Sergio Martin, Kazushi Sakamoto, Sebastien Muller, Kunihiko Tanaka, Laura Colzi, Christian Henkel, David S. Meier, Victor M. Rivilla, Paul P. van der Werf
Subjects: Astrophysics of Galaxies (astro-ph.GA)
Cite as: arXiv:2409.13821 [astro-ph.GA] (or arXiv:2409.13821v1 [astro-ph.GA] for this version)
https://doi.org/10.48550/arXiv.2409.13821
Focus to learn more
Submission history
From: Erica Behrens
[v1] Fri, 20 Sep 2024 18:01:41 UTC (10,918 KB)
https://arxiv.org/abs/2409.13821
Astrobiology, Astrochemistry,