Estimating Exoplanet Mass Using Machine Learning on Incomplete Datasets
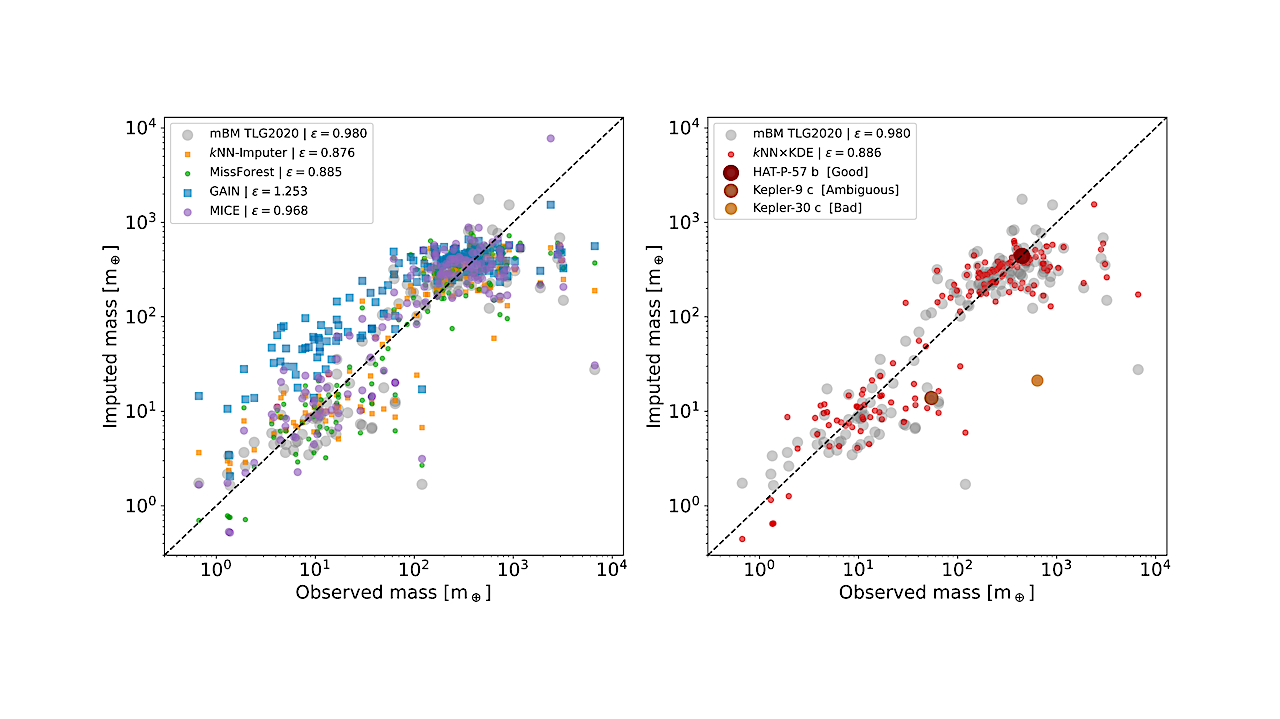
The exoplanet archive is an incredible resource of information on the properties of discovered extrasolar planets, but statistical analysis has been limited by the number of missing values. One of the most informative bulk properties is planet mass, which is particularly challenging to measure with more than 70% of discovered planets with no measured value.
We compare the capabilities of five different machine learning algorithms that can utilize multidimensional incomplete datasets to estimate missing properties for imputing planet mass. The results are compared when using a partial subset of the archive with a complete set of six planet properties, and where all planet discoveries are leveraged in an incomplete set of six and eight planet properties.
We find that imputation results improve with more data even when the additional data is incomplete, and allows a mass prediction for any planet regardless of which properties are known. Our favored algorithm is the newly developed kNN×KDE, which can return a probability distribution for the imputed properties. The shape of this distribution can indicate the algorithm’s level of confidence, and also inform on the underlying demographics of the exoplanet population.
We demonstrate how the distributions can be interpreted with a series of examples for planets where the discovery was made with either the transit method, or radial velocity method. Finally, we test the generative capability of the kNN×KDE to create a large synthetic population of planets based on the archive, and identify potential categories of planets from groups of properties in the multidimensional space. All codes are Open Source.
Florian Lalande, Elizabeth Tasker, Kenji Doya
Comments: 30 pages, 14 figures, 1 table. Accepted for publication in the Open Journal of Astrophysics
Subjects: Earth and Planetary Astrophysics (astro-ph.EP); Instrumentation and Methods for Astrophysics (astro-ph.IM); Machine Learning (cs.LG)
Cite as: arXiv:2410.06922 [astro-ph.EP] (or arXiv:2410.06922v1 [astro-ph.EP] for this version)
https://doi.org/10.48550/arXiv.2410.06922
Focus to learn more
Submission history
From: Florian Lalande
[v1] Wed, 9 Oct 2024 14:19:33 UTC (4,942 KB)
https://arxiv.org/abs/2410.06922
Astrobiology, Astronomy,