Advancing Machine Learning for Stellar Activity and Exoplanet Period Rotation
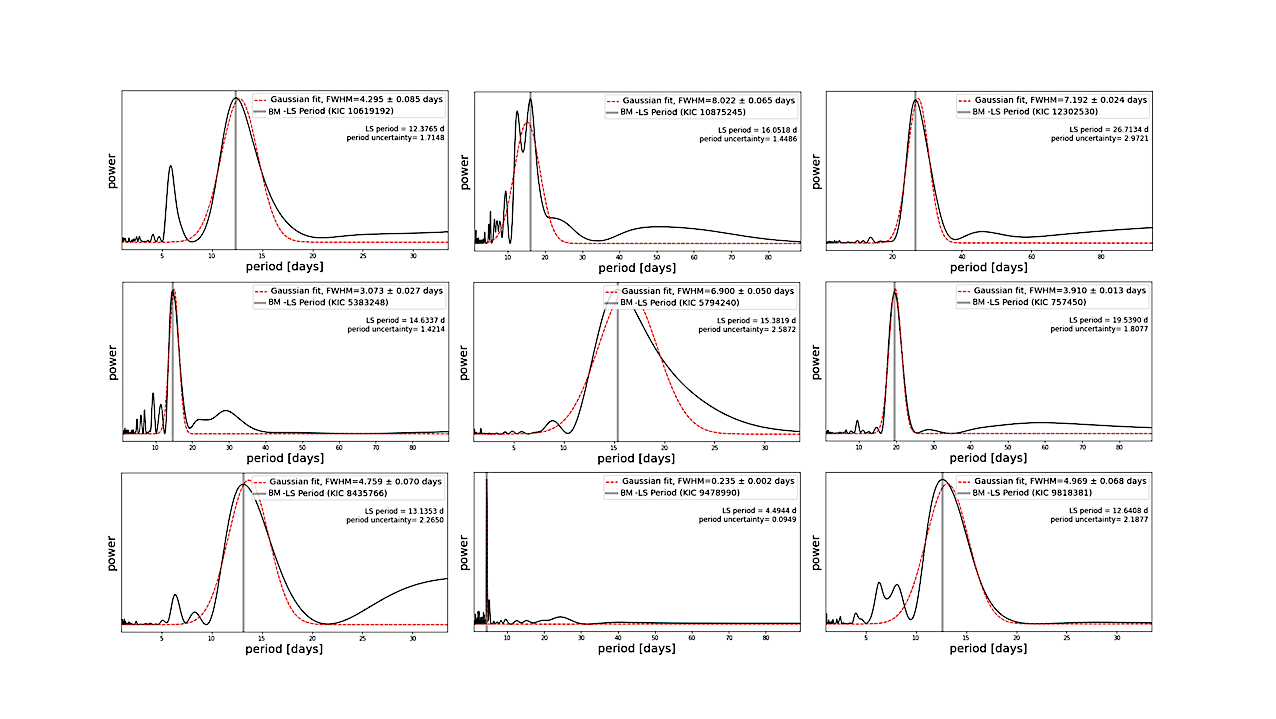
This study applied machine learning models to estimate stellar rotation periods from corrected light curve data obtained by the NASA Kepler mission. Traditional methods often struggle to estimate rotation periods accurately due to noise and variability in the light curve data.
The workflow involved using initial period estimates from the LS-Periodogram and Transit Least Squares techniques, followed by splitting the data into training, validation, and testing sets. We employed several machine learning algorithms, including Decision Tree, Random Forest, K-Nearest Neighbors, and Gradient Boosting, and also utilized a Voting Ensemble approach to improve prediction accuracy and robustness.
The analysis included data from multiple Kepler IDs, providing detailed metrics on orbital periods and planet radii. Performance evaluation showed that the Voting Ensemble model yielded the most accurate results, with an RMSE approximately 50% lower than the Decision Tree model and 17% better than the K-Nearest Neighbors model.
The Random Forest model performed comparably to the Voting Ensemble, indicating high accuracy. In contrast, the Gradient Boosting model exhibited a worse RMSE compared to the other approaches. Comparisons of the predicted rotation periods to the photometric reference periods showed close alignment, suggesting the machine learning models achieved high prediction accuracy.
The results indicate that machine learning, particularly ensemble methods, can effectively solve the problem of accurately estimating stellar rotation periods, with significant implications for advancing the study of exoplanets and stellar astrophysics.
Fatemeh Fazel Hesar, Bernard Foing, Ana M. Heras, Mojtaba Raouf, Victoria Foing, Shima Javanmardi, Fons J. Verbeek
Comments: 15 pages, 8 figures. Submitted for publication in A&A
Subjects: Solar and Stellar Astrophysics (astro-ph.SR); Earth and Planetary Astrophysics (astro-ph.EP); Instrumentation and Methods for Astrophysics (astro-ph.IM); Machine Learning (cs.LG)
Cite as: arXiv:2409.05482 [astro-ph.SR] (or arXiv:2409.05482v1 [astro-ph.SR] for this version)
https://doi.org/10.48550/arXiv.2409.05482
Focus to learn more
Submission history
From: Fatemeh Fazel Hesar
[v1] Mon, 9 Sep 2024 10:25:13 UTC (2,436 KB)
https://arxiv.org/abs/2409.05482
Astrobiology