A Statistical And Machine Learning Approach To The Study Of Astrochemistry
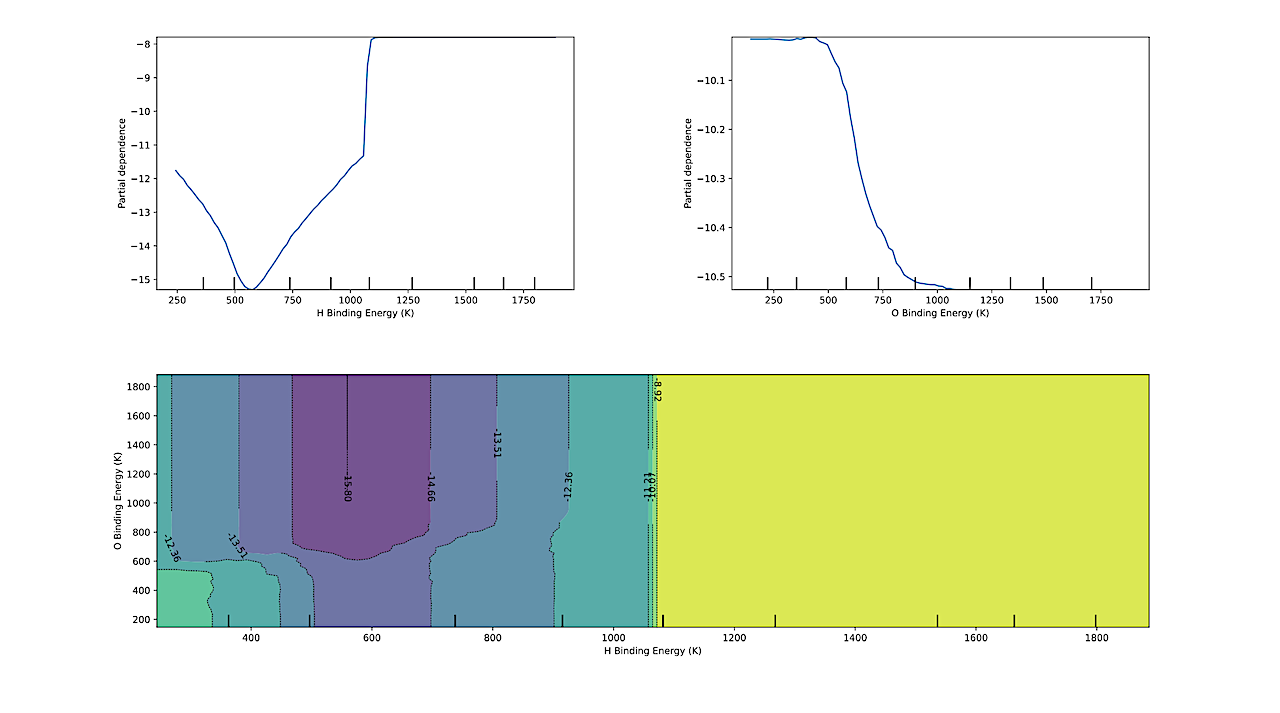
In order to obtain a good understanding of astrochemistry, it is crucial to better understand the key parameters that govern grain-surface chemistry. For many chemical networks, these crucial parameters are the binding energies of the species.
However, there exists much disagreement regarding these values in the literature. In this work, a Bayesian inference approach is taken to estimate these values. It is found that this is difficult to do in the absence of enough data. The Massive Optimised Parameter Estimation and Data (MOPED) compression algorithm is then used to help determine which species should be prioritised for future detections in order to better constrain the values of binding energies.
Finally, an interpretable machine learning approach is taken in order to better understand the non-linear relationship between binding energies and the final abundances of specific species of interest.
Comments: Accepted for publication in Faraday Discussions 2023. 14 pages, 7 figures and 1 table Subjects: Astrophysics of Galaxies (astro-ph.GA) Cite as:arXiv:2306.05790 [astro-ph.GA] (or arXiv:2306.05790v1 [astro-ph.GA] for this version)
Johannes Heyl, Serena Viti, Gijs Vermariën
https://doi.org/10.48550/arXiv.2306.05790
Focus to learn more Submission history From: Johannes Heyl
[v1] Fri, 9 Jun 2023 10:07:10 UTC (1,960 KB)
https://arxiv.org/abs/2306.05790
Astrobiology, Astrochemistry