Constraining Chemical Networks In Astrochemistry
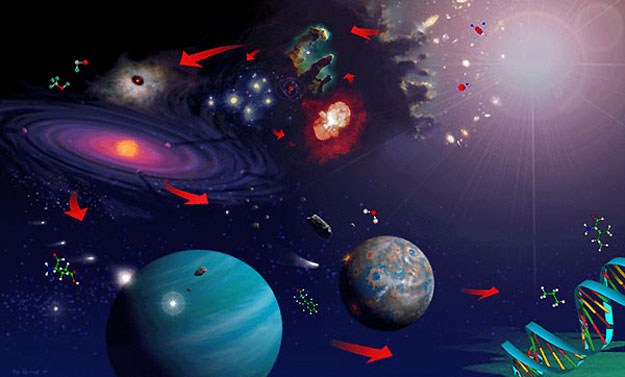
Databases of gas and surface chemical reactions are a key tool for scientists working in a wide range of physical sciences. In Astrochemistry, databases of chemical reactions are used as inputs to chemical models to determine the abundances of the interstellar medium.
Gas chemistry and, in particular, grain surface chemistry and its treatment in gas-grain chemical models are however areas of large uncertainty. Many reactions – especially on the dust grains – have not been systematically experimentally studied. Moreover, experimental measurements are often not easily translated to the rate equation approach most commonly used in astrochemical modelling.
Reducing the degree of uncertainty intrinsic in these databases is therefore a prime problem, but has so far been approached mainly by ad hoc procedures of essentially trial and error. In this chapter we review the problem of the determination of accurate and complete chemical networks in the wider context of Astrochemistry and explore the possibility of using statistical methods and machine learning (ML) techniques to reduce the uncertainty in chemical networks.
Serena Viti, Jonathan Holdship
Comments: Published as a chapter in “Machine Learning in Chemistry: The Impact of Artificial Intelligence”
Subjects: Astrophysics of Galaxies (astro-ph.GA)
DOI: 10.1039/9781839160233
Cite as: arXiv:2101.05174 [astro-ph.GA] (or arXiv:2101.05174v1 [astro-ph.GA] for this version)
Submission history
From: Jonathan Holdship
[v1] Wed, 13 Jan 2021 16:24:45 UTC (50 KB)
https://arxiv.org/abs/2101.05174
Astrobiology, astrochemistry,